Application of tNGS in Pathogen Detection Analysis for Clinical Samples
View: 3731 / Time: 2023-12-13
Background
Metagenomic Next-Generation Sequencing (mNGS) has emerged as an attractive strategy for unbiased and comprehensive microbial detection and taxonomic characterization. Despite its success in improving the diagnosis, treatment, and tracking of infectious diseases, mNGS faces challenges in terms of detection sensitivity and cost[1-2]. Targeted Next-Generation Sequencing (tNGS) offers advantages in enriching specific pathogens or groups of pathogens, as well as other genes of interest. tNGS significantly reduces the amount of raw sequencing data while increasing the information on pathogenic microorganisms in samples and improving sensitivity, achieving a dual optimization of performance and cost. Therefore, mNGS is more suitable for scenarios involving unpredictable pathogens, while tNGS is more suitable for situations requiring higher sensitivity. Compared to mNGS, tNGS pathogen detection has the following technical advantages[3-5]:
1. Less susceptible to interference from the human genome and background microbiota.
2. More sensitive for detecting RNA viruses, intracellular bacteria, and engulfed pathogens.
3. Direct detection of phenotype-related genes, such as drug resistance and virulence genes.
4. Real quantitative detection of pathogens.
5. Cost-effective detection.
Recently, Nanodigmbio launched a new tNGS solution based on probe hybridization capture called NEX-t Panel (New Product Launch | NEX-t Panel: a more precise and cost-effective tNGS pathogen detection solution). This solution targets a series of characteristic sequences selected from the genomes of hundreds of pathogens, including viruses, bacteria, fungi, and parasites. Additionally, it covers regions such as 16S/ITS, housekeeping genes, and antibiotic resistance-related genes. The accompanying one-stop bioinformatics analysis tool, NEX-tScan, is applicable for pathogen microbiome detection analysis and full-length capture detection analysis of 16S and ITS. In this article, we primarily showcase its performance in the detection of microbial standard samples and clinical samples, comparing it with mNGS and multiplex PCR validation.
Materials and Methods
Sample Sources
Microbial Standards: A mixture of 20 strains of genomic material (ATCC, MSA-1003), with 13 microbial genomes are designed for the coverage in NEX-t Panel v1.0, all 20 microbial genomes are covered by 16S full-length probes. The standards mentioned above were gradient diluted with human genomic DNA standard (Promega, G1471) to obtain four samples with concentrations of 1%, 0.1%, 0.01%, and 0.001% for testing.
Clinical Samples: 21 clinical patient samples, all collected from patients diagnosed with potential pathogen infections. The sample types include tissues, urine, blood, pus, wounds, cerebrospinal fluid, bronchoalveolar lavage fluid, sputum, uterine secretions, and unknown sources.
Experimental Procedure
All samples were processed using the Nanodigmbio NadPrep EZ DNA Library Preparation Kit v2 to prepare pre-libraries. Subsequently, NadPrep ES Hybrid Capture Reagents and NEX-t Panel v1.0 were utilized for capture, followed by PE150 sequencing on the Illumina platform. To simulate common SE50 and SE100 rapid sequencing strategies often used in pathogen detection, we created SE100 and SE50 datasets by trimming read lengths from the original sequencing data. Subsequently, comparative analyses were performed on datasets with different read lengths. For the 21 clinical samples, DNA samples from the same batch were parallelly processed by third parties for multiplex PCR sequencing (SE60) and mNGS (SE100) respectively. Comparative analyses were conducted with the respective pathogen detection reports.
NEX-tScan Pathogen Database
Bulit upon the design strategy of NEX-t Panel, NEX-tScan constructs f an internal pathogen database by aligning scoring captured species sequences through an internal matching algorithm and comparisons with public databases. Each pathogen in the database has been annotated, categorized and manually reviewed. Annotations include bacteria, fungi, viruses, parasites, mycoplasma/chlamydia, and special pathogens (Mycobacterium tuberculosis complex) among others. Additionally, the database classifies pathogens based on public databases and literature reports, including common pathogens, common environmental background microbes, colonizing microbes, etc., providing references for subsequent analysis and reporting.
Results
Microbial Standards
In the microbial standards with concentrations of 1% and 0.1%, analysis using three sequencing modes, PE150, SE100, and SE50, showed that NEX-t Panel achieved 100% detection of 13 microbial species, and the amount of captured data exhibited a correlation with the proportion of each microorganism with an R2 value > 0.7 (Figure 1. A&B). Furthermore, at both concentrations, 16S-targeted sequencing data alone detected all 20 microorganisms, with R2 correlation > 0.7 (Figure 1. C&D). However, in the 0.01% and 0.001% concentration microbial standards, due to the theoretical copy number of some species (Figure 1. E) being close to 0, they could not be detected, resulting in a reduced detection rate (Figure 1. A&C). The overall analysis of microbial standards across a concentration gradient of 0.001% to 1% shows that microorganisms were effectively detected even when the microbial content was as low as 3 copies.
Although there were no significant differences observed in the detection results among the three data analysis modes of PE150, SE100, and SE50, there are significant differences in the amount of captured data. As shown in Figure 2, due to more precise alignment, the number of reads supporting detection in PE150 and SE100 modes is significantly higher than in SE50.
Figure 1. Detection rate and correlation analysis of gradient microbial standard samples using targeted capture and 16S capture. A. Detection rate of specifically captured species; B. Correlation between the number of reads of specifically captured species and standard concentration; C. Detection rate of 16S species; D. Correlation between the number of reads of 16S species and standard concentration; E. Theoretical copy number of microbial standard.
Figure 2. Detected the No. of supporting reads (logarithm with a base of 10) of the gradient microbial standards under different sequencing analysis modes. A. Heatmap of the amountof targeted capture for 13 bacterial species. B. Heatmap of the amount of 16S region capture for 20 bacterial species.
Clinical Sample
Detection Results of Different Technical Approaches
We initially analyzed and compared the results of three technical approaches: NadPrep hybrid capture (NEX-t Panel, SE50), multiplex PCR amplification, and mNGS applied to the detection of 21 clinical samples. To eliminate interfering factors, all analyses were based on the same sample with clinically unknown characteristics but a clearly identified tissue sources. At the same time, we employed the strictest criteria for assessment: reporting the pathogenic species completely in line with the "reference result" was considered correct, while under-reporting, over-reporting, and mis-reporting were all deemed as errors (Table 1).
Among the 21 clinical samples, the accuracy rates were as follows: multiplex PCR amplification achieved 47.6% accuracy (10/21), the mNGS achieved an accuracy of 61.9% (13/21), and Hybrid Capture achieved 71.4% accuracy (15/21). Specifically, multiplex PCR amplification had 7 cases of false-positive errors due to mis-reporting and 4 cases of false-negative errors due to under-reporting; the mNGS had 4 cases of false-positive errors, 1 case of false-negative error, and 3 cases of complete errors; while the hybrid capture had 5 cases of false-positive errors and 1 case of false-negative error.
Table 1. Pathogen detection analysis results using different technical approaches.
Figure 3. Summary of pathogen detection analysis results using different technical approaches.
Among the 7 false-positive errors from the multiplex PCR amplification, nearly half (3 out of 7) were due to the over-reporting of Human alphaherpesvirus 1, which we suspect may be due to contamination during the operation process or defects in the product itself. Among the 4 false-negative errors, the inability to detect Corynebacterium jeikeium in sample 6 and Finegoldia magna in sample 7 might be due to the absence of the corresponding species in the PCR product, indirectly reflecting the limitations of PCR amplification in detecting unconventional pathogens.
The false positive errors in the mNGS analysis were more scattered, without concentrated over-reporting of any particular species. The 1 false-negative error and 3 complete errors were due to either the lack of particularly advantageous species in the data or errors in the analysis caused by a low number of supporting reads, this reflects the common challenge in mNGS data analysis, where limited effective data can lead to difficulties in interpretation.
All 5 false positive errors in the hybrid capture were concentrated in bacteria groups with high homogeneity, such as Klebsiella, Salmonella, and Escherichia coli . This was caused by the broad-spectrum alignment resulting from the short sequencing reads and the wide-ranging coverage of Nex-t Panel. In the case of the false-negative errors in sample 8, Streptococcus pneumoniae was missed, likely because its proportion was closer to the background bacteria. It is noteworthy that in sample 7, the detection of Finegoldia magna was successfully despite the absence of specific capture probes, likely because the NEX-t Panel v1.0 includes 16S probes enabling its detection.
In general, conventional pathogen detection with multiplex PCR amplification may introduce some risk of false negatives due to the limitations in primer design . In the case of the mNGS poses challenges in effective data control and analysis complexity, leading to difficulties in discerning false positives or false negatives, . Hybrid capture, compared to the former, exhibits a broader capabilities in detecting various pathogens, and enriches more effective data compared to the latter. In clinical applications. Consequently, its performance in detecting false positives and false negatives surpasses both. In clinical applications, the preliminary results from all three technical approaches should be further verified by experienced physicians, considering specific clinical manifestations, to generate conclusive reports.
Further Improvement in Accuracy
To compare the impact of different sequencing read lengths on pathogen detection, we focused on evaluating the analysis results of the hybrid capture under three data modes: SE50, SE100, and PE150. Overall, the detection of microbial species in various samples is generally consistent across different sequencing read lengths, indicating that the length of the reads captured with NEX-t Panel v1.0 has limited influence on the detection of pathogenic microorganisms.
Samples 16 and 19 exhibited significant false-positive detection in the SE50 mode. However, in both SE100 and PE150 modes, the detection results were completely consistent with the reference results (Table 2). This suggests that the longer sequencing read length enhance the ability to distinguish highly homologous species is higher, reducing the occurrence of false-positive and thereby improving the accuracy of the results.
Although the false-positive detections in the other three other samples (sample 3, 4, and 20) did not disappear with the increased in read length, they were all due to the ambiguous classification with in Enterobacteriaceae family, such as Klebsiella, with other highly homologous bacteria. In reality, species with high homology, such as Shigella and Escherichia coli, often require interpreters to make comprehensive judgments and interpretations based on species abundance, supporting reads counts, and clinical phenotypes.
Table 2. Comparison of analysis results with different length of sequencing reads.
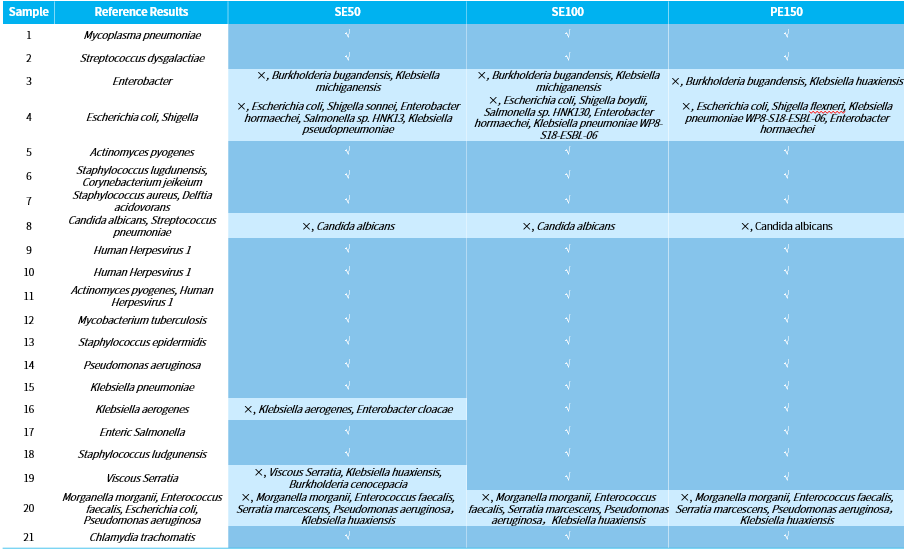
Discussion
When clinical samples contain complex host and environmentally diverse backgrounds, mNGS may be prohibitively expensive and may even be impractical for the direct detection of pathogenic microorganisms through NGS or traditional molecular techniques. Hybrid capture, which are cost-effective, versatile, and platform-independent, can significantly minimizes the cost of microbial genome sequencing in most cases and reliably elucidate microbial strain sequences, gene content, and genome structures. When applied to multi-strain identification, virulence detection, tracking history transmission, or elucidating species evolution, hybrid capture offer unmatched efficiency in microbial genome sequencing compared to other techniques [6-7].
Both hybrid capture and multiplex PCR amplification can effectively enrich known sequences, but only hybrid capture with probes can enrich sequences that have undergone significant recombination variations or SNP-Indel mutations, such as rapidly mutating sequences found in various new strains of COVID-19. The NEX-t Panel v1.0 introduced by Nanodigmbio is a more cost-effective and convenient solution compared to large-scale hybrid capture tNGS panels available in the market. Although NEX-t Panel v1.0 also has limitations in terms of the range of pathogen detection, due to the excellent scalability of hybrid capture with probes, the detection scope can be further expanded based on the actual clinical detection requirements by adding probes.
[1] Dahyot S, Lemee L, Pestel-Caron M. Description et place des techniques bactériologiques dans la prise en charge des infections pulmonaires [J]. Rev Mal Respir. 2017;34(10):1098-1113.
[2] Ju C R, Lian Q Y, Guan W J, et al. Metagenomic next-generation sequencing for diagnosing infections in lung transplant recipients: a retrospective study[J]. Transplant International, 2022, 35: 10265.Gaston, D. C. et al. Journal of clinical microbiology 60, 7(2022).
[3] Gaston D C, Miller H B, Fissel J A, et al. Evaluation of metagenomic and targeted next-generation sequencing workflows for detection of respiratory pathogens from bronchoalveolar lavage fluid specimens[J]. Journal of clinical microbiology, 2022, 60(7): e00526-22.
[4] Campana M G, Hawkins M T R, Henson L H, et al. Simultaneous identification of host, ectoparasite and pathogen DNA via in‐solution capture[J]. Molecular Ecology Resources, 2016, 16(5): 1224-1239.
[5] Duggan A T, Perdomo M F, Piombino-Mascali D, et al. 17th century variola virus reveals the recent history of smallpox[J]. Current Biology, 2016, 26(24): 3407-3412Forth, J.H. et al. (2019) A Deep-Sequencing Workflow for the Fast and Efficient Generation of High-Quality African Swine Fever Virus Whole-Genome Sequences. Viruses.
[6] Forth J H, Forth L F, King J, et al. A deep-sequencing workflow for the fast and efficient generation of high-quality African swine fever virus whole-genome sequences[J]. Viruses, 2019, 11(9): 846.
[7] Vezzulli L, Grande C, Tassistro G, et al. Whole-genome enrichment provides deep insights into Vibrio cholerae metagenome from an African river[J]. Microbial ecology, 2017, 73: 734-738.